Hi everyone-
The news from Ukraine is truly devastating and brings a huge dose of perspective to our day to day lives in the UK. I know I for one care rather less about fixing my python package dependencies when I see the shocking scenes from Mariupol… However, those of us more distant from the war do at least have the option to think about other things, and hopefully the data science reading materials below might distract a little…
Following is the April edition of our Royal Statistical Society Data Science and AI Section newsletter. Hopefully some interesting topics and titbits to feed your data science curiosity. Check out our new ‘Jobs!’ section… an extra incentive to read to the end!
As always- any and all feedback most welcome! If you like these, do please send on to your friends- we are looking to build a strong community of data science practitioners. And if you are not signed up to receive these automatically you can do so here.
Industrial Strength Data Science April 2022 Newsletter
RSS Data Science Section
Committee Activities
We have all been shocked and saddened by events in the Ukraine and our thoughts and best wishes go out to everyone affected
The committee is busy planning out our activities for the year with lots of exciting events and even hopefully some in-person socialising… Watch this space for upcoming announcements.
Louisa Nolan (Chief Data Scientist, Data Science Campus, ONS) is helping drive the Government Data Science Festival 2022, a virtual event running from 27 April to 11 May 2022. This exciting event is a space for the government and UK public sector data science community, and colleagues in the academic sector, to come together to learn, discover, share and connect. This year’s theme is: The Future of Data Science for Public Good. Register here!
Anyone interested in presenting their latest developments and research at the Royal Statistical Society Conference? The organisers of this year’s event – which will take place in Aberdeen from 12-15 September – are calling for submissions for 20-minute and rapid-fire 5-minute talks to include on the programme. Submissions are welcome on any topic related to data science and statistics. Full details can be found here. The deadline for submissions is 5 April.
Janet Bastiman (Chief Data Scientist at NapierAI) recorded a podcast with Moodys on “AI and transparent boxes”, looking at the use of AI in detecting financial crime and explainability- will post the link once it is published.
Giles Pavey (Global Director Data Science at Unilever) was interviewed for the Data Storytellers podcast about his career in data science – check it out here.
Martin Goodson (CEO and Chief Scientist at Evolution AI) continues to run the excellent London Machine Learning meetup and is very active with events. The next event is on April 13th when Martha White, (Associate Professor of Computing Science at the University of Alberta), discusses her research on “Advances in Value Estimation in Reinforcement Learning“. Videos are posted on the meetup youtube channel – and future events will be posted here.
As we highlight in the Members and Contributors section, Martin was interviewed by the American Statistical Association (ASA) about Practical Data Science & The UK’s AI Roadmap
This Month in Data Science
Lots of exciting data science going on, as always!
Ethics and more ethics…
Bias, ethics and diversity continue to be hot topics in data science…
- The war in the Ukraine is front page news across the world. The conflict is being fought in new ways, with drones playing key roles. Not surprisingly, this highlights the increasing influence of AI in warfare and the potential implications
"The notion of a killer robot—where you have artificial intelligence fused with weapons—that technology is here, and it's being used,” says Zachary Kallenborn, a research affiliate with the National Consortium for the Study of Terrorism and Responses to Terrorism (START).
- Separately, DeepFakes are also playing their part- a Deep Fake of Ukrainian President Zelensky apparently ordering his troops to surrender was uploaded to Facebook and YouTube and circulated widely, but the Ukrainian Government was quickly able to issue a response.
That short-lived saga could be the first weaponized use of deepfakes during an armed conflict, although it is unclear who created and distributed the video and with what motive. The way the fakery unraveled so quickly shows how malicious deepfakes can be defeated—at least when conditions are right.
Not all people targeted by deepfakes will be able to react as nimbly as Zelensky—or find their repudiation so widely trusted. “Ukraine was well positioned to do this,” Gregory says. “This is very different from other cases, where even a poorly made deepfake can create uncertainty about authenticity.”
- A version of a DeepFake was used controversially in the recent South Korean Presidential Elections, where one of the candidates successfully created an AI version of himself…
While debates are heating up on AI campaigning, the National Election Commission (NEC) is yet to determine whether it is legitimate or not. "It is difficult to make a finding on whether it is against the laws governing campaigning or not because it is uncertain how the technologies will be used in the campaign," an NEC official said.
- However, uncertainty over the ethics and efficacy of facial recognition systems is not stopping the controversial provider, ClearViewAI, from making commercial progress with many leading law enforcement agencies in the US. Indeed, the system is apparently being used in the Ukraine to identify dead Russian soldiers.
- Similar image recognition techniques underly recent breakthroughs in diagnosing rare genetic disorders from facial characteristics, a capability that is already being commercialised.
- We have previously talked about the in-built racial and socio-economic biases encoded in the data used to train some publicly used AI systems, but potential biases are not limited to those dimensions. Recent research published by the WHO highlights examples of agism in AI enabled healthcare services.
- A recent ruling from the FTC in the US is demanding that Weight Watchers “destroy the algorithms or AI models it built using personal information collected through its Kurbo healthy eating app from kids as young as 8 without parental permission”. Interesting development, but not entirely sure what it means and how you would implement it- could you simply retrain the model on new data?
- It is becoming easier and easier to create AI systems. There are now a number of solutions available that allow anyone to feed in data and generate automated AI models without writing a single line of code. While there are clearly benefits to increasing access, particularly for well defined use-cases…
Just as clickable icons have replaced obscure programming commands on home computers, new no-code platforms replace programming languages with simple and familiar web interfaces. And a wave of start-ups is bringing the power of A.I. to nontechnical people in visual, textual and audio domains.
… there are also obvious downsides, with the increased risk of miss-application a key one…
“If you’re using low-code, no-code, you don’t really have a good sense of the quality of the ingredients coming in, and you don’t have a sense of the quality of the output either,” he said. While low- and no-code software have value for use in training or experimentation, “I just wouldn’t apply it in subject areas where the accuracy is paramount”.
- We have looked into recommendation systems in the past, and how more ‘provocative’ information tends to spread more widely than more mundane (but accurate) news (interesting take on TikTok and the Ukraine War here) – new research using data from Weibo in China gives more insight.
Surprisingly, we find that anger travels easily along weaker ties than joy, meaning that it can infiltrate different communities and break free of local traps because strangers share such content more often
- The Stanford University HAI 2022 AI Index report is out, packed with excellent information and insight. The Global AI Vibrancy tool highlights which countries are making the most advances, with US and China leading the way, not surprisingly. The Brookings Institute has published interesting research highlighting how this AI Leadership has been driven by underlying increases in available computing power
- Somewhat ironically, Meta (Facebook) has announced what looks to be a promising approach to building AI systems while protecting privacy In addition they are apparently “committed to Transparency and Control as one of our five pillars of Responsible AI“
- Finally an interesting take on the use of AI based provenance in art history, from an art historian…
When AI gets attention for recovering lost works of art, it makes the technology sound a lot less scary than when it garners headlines for creating deep fakes that falsify politicians’ speech or for using facial recognition for authoritarian surveillance.
Developments in Data Science…
As always, lots of new developments on the research front and plenty of arXiv papers to read…
- When the great Yoshua Bengio speaks, it’s normally worth listening… Generative Flow Networks
"I have rarely been as enthusiastic about a new research direction. We call them GFlowNets, for Generative Flow Networks. They live somewhere at the intersection of reinforcement learning, deep generative models and energy-based probabilistic modelling"
- While large models get ever larger, and our computational capabilities get ever greater (“AI computer maker Graphcore unveils 3-D chip, promises 500-trillion-parameter ‘ultra-intelligence’ machine“), researchers are always on the lookout for efficiency, and this seems like a great win: “HPC-AI’s FastFold Shortens AlphaFold Training Time from 11 Days to 67 Hours“, and fantastic that they have published their code
- When models become so large, it becomes incredible difficult and time consuming to tune their hyper-parameters. Microsoft has has released µTransfer, a new approach to doing this
"µP provides an impressive step toward removing some of the black magic from scaling up neural networks. It also provides a theoretically backed explanation of some tricks used by past work, like the T5 model. I believe both practitioners and researchers alike will find this work valuable."
- Ai Explainability continues to be a hot research topic. Most widely used approaches attempt to ‘explain’ a given AI output by approximating the local decision criteria. ‘CX-TOM‘ looks to be an interesting new approach in which it “generates sequence of explanations in a dialog by mediating the differences between the minds of machine and human user”
- Speaking of ‘minds’ … useful summary of recent Neuroscience/ML research
Reading and being aware of the evolution and new insights in neuroscience not only will allow you to be a better “Artificial Intelligence” guy 😎, but also a finer neural network architectures creator 👩💻!
- Comprehending images and videos is something we all take for granted as humans. However it is an incredible complex task for AI systems, and although we have got a lot better in recent years, even the best systems can still be easily led astray. So research continues, particularly in understanding actions and processes:
- Google has made progress in recognising actions by co-training their transformer models with both images and videos
- And Google has also made significant progress in leveraging these insights into practical tools
- Researchers from Stanford, USC, Microsoft and Google have created video2sim which generates a simulation from a video of an articulated mechanism
- Even with the breakthroughs of GPT-3 and other large language models, comprehension and trust (almost “common sense”) are still huge challenges in natural language processing as well. Researchers at DeepMind have released GopherCite which adds a bit more “sense” to the responses given to factual questions (great quote below… emphasis mine!)
“Recent large language models often answer factual questions correctly. But users can't trust any given claim a model makes without fact-checking, because language models can hallucinate convincing nonsense. In this work we use reinforcement learning from human preferences (RLHP) to train "open-book" QA models that generate answers whilst also citing specific evidence for their claims, which aids in the appraisal of correctness"
- This sounds quite arcane (“Your Policy Regulariser is Secretly an Adversary“) but when Shane Legg, one of the founding fathers at DeepMind, is involved it’s worth reading. It does feel quite profound:
The standard model for sequential decision-making under uncertainty is the Markov decision process (MDP). It assumes that actions are under control of the agent, whereas outcomes produced by the environment are random ... This, famously, leads to deterministic policies which are brittle — they “put all eggs in one basket”. If we use such a policy in a situation where the transition dynamics or the rewards are different from the training environment, it will often generalise poorly.
We want to train a policy that works well, even in the worst-case given our uncertainty. To achieve this, we model the environment to not be simply random, but being (partly) controlled by an adversary that tries to anticipate our agent’s behaviour and pick the worst-case outcomes accordingly.
- Better generalisation in our models is something we all strive for. When we know we have training data that fully encompasses the ‘field of use’ for the AI system, we can be confident in the model generalisation, but this is often not the case. Stock markets are. a case in point (and the failing of many a brilliant “backtested” quant trading strategy…) so research into more advanced simulation of potential ‘futures’ (using GANs) to use in testing is intriguing.
- Finally another take on assessing generalisation which I think could be groundbreaking: “Assessing Generalization of SGD via Disagreement“, from researchers at Carnegie Mellon University, which allows you assess generalised errors using unlabelled data only!
Stochastic gradient descent (SGD) is perhaps the most popular optimization algorithm for deep neural networks. Due to the non-convex nature of the deep neural network’s optimization landscape, different runs of SGD will find different solutions. As a result, if the solutions are not perfect, they will disagree with each other on some of the unseen data. This disagreement can be harnessed to estimate generalization error without labels:
1) Given a model, run SGD with the same hyperparameters but different random seeds on the training data to get two different solutions.
2) Measure how often the networks’ predictions disagree on a new unlabeled test dataset.

Real world applications of Data Science
Lots of practical examples making a difference in the real world this month!
- AI approaches continue to break new ground in medicine and healthcare:
- What sounds like good progress in predicting gene expression from DNA sequences
- Understanding how psychedelic drugs affect the brain… using NLP to diagnose “trip reports”!
- Useful meta analysis from Stanford HAI on how machine learning is helping us understand how the brain works
This analysis showed that different parts of the brain work together in surprising ways that differ from current neuroscientific wisdom. In particular, the study calls into question our current understanding of how brains process emotion
- Differing takes on using AI/optimisation techniques to improve healthcare access and costs in the US:
- Not sure what I think of this… “Pig grunts reveal their emotions“..
- Casinos attempting to help stop gambling addiction with AI.. Good balanced article from the NYTimes highlighting the potential but also the conflict of interest between gambler and casino
“Some operators have more robust responsible gambling programs than others,” says Lia Nower, director of the Center for Gambling Studies at Rutgers University. “But in the end there is a profit motive and I have yet to see an operator in the U.S. put the same amount of money and effort into developing a system for identifying and assisting at-risk players as they do developing A.I. technologies for marketing or extending credit to encourage players to return.”
- Nowcasting is useful concept in the modern world – how can make the most of whatever information is currently available to understand the state of the world now or in the near future. Good progress in near-time precipitation forecasting. (“Alexa, should I bring an umbrella?” … “I don’t know, let me check my DGMR PySteps model”…)
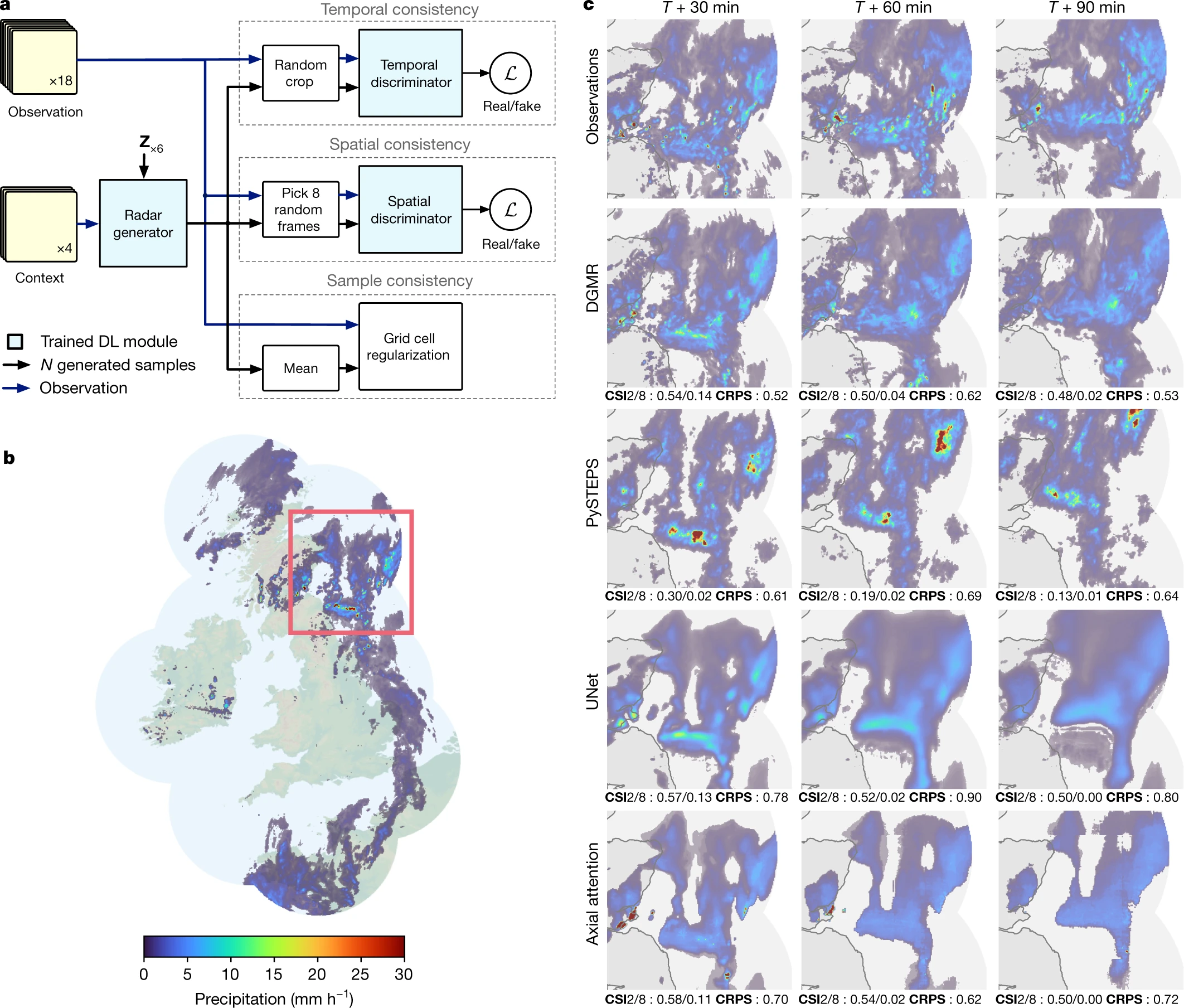
- A bit light on details, but MIT-IBM Watson AI Lab have released a new approach to anomaly detection in large and complex networks, such as power-grids. Lots of great applications if it works in the real world…
- Apparently the “Great Resignation” has driven the adoption lots of AI based automation systems and applications
- There is still much debate about how and when self driving cars will take over our roads. A new approach to robot navigation from UC Berkeley might help…
- Lots going on in the world on NLP and language applications … starting with Facebook/Meta who are doubling down on creating a “universal speech translator“. Love the understated sub-title: “Universal AI translation could be a killer app for Meta’s future“…
- Google is now automating document summarisation within Google Docs …. which may put a few “cliff notes” companies out of business… Again, Google are very impressive at taking cutting edge research (large pre-trained language models for both natural language understanding and natural language understanding) and embedding it in practical tools available to anyone
- Perhaps as a counterpoint to the slightly grumpy art-historian we mentioned in the section above, DeepMind is using its formidable capabilities to help historians better interpret ancient inscriptions– not just translating, but attribution both chronologically and geographically.
- An in-depth investigative piece from the NYTimes on identifying previously anonymous QAnon posters from linguistic characteristics using ML techniques
Instead of relying on expert opinion, the computer scientists used a mathematical approach known as stylometry. Practitioners say they have replaced the art of the older studies with a new form of science, yielding results that are measurable, consistent and replicable.
- Finally, taking the ‘auto-coders’ out for a spin…
- Writing games with ‘no-code’ (including a minimal ‘Legend of Zelda’) using OpenAI’s code-davinci codex model. “There are limitations, and coding purely by simple text instructions can stretch your imagination, but it’s a huge leap forward and a fun experiment”
- Meanwhile researchers at Carnegie Mellon University have released ‘Polycoder‘ which they claim is even better
- And now that ‘Copilot’ (powered by OpenAI codex) has been in the wild for a little while, we are starting to see interesting use cases and commentary
"I too was pretty skeptical of Copilot when I started using it last summer.
However it is shockingly good for filling out Python snippets - ie smarter autocomplete when teaching.
Popular libraries like Pandas, Beautiful Soup, Flask are perfect for this.
About 80% time it will fill out the code exactly they way I would want. About 10% time it will be something you want to correct or nudge.
Then about 10% of time it will be a howler or anti-pattern."
How does that work?
A new section on understanding different approaches and techniques
- Back to basics- a lovely primer on probability from none other than Peter Norvig (and lots more here, a really excellent resource )
- Sentiment analysis is a useful tool in the toolkit – good primer from Sultan Al Awar walking through 2 different approaches (scikit vs keras)
- Going deeper into language processing- this is an excellent tutorial on NLP embeddings
I still struggle with the basic 4 dimensions of our physical world. When I first heard about 768-dimension embeddings, I feared my brain would escape from my ear. If you can relate, if you want to truly master the tricky subject of NLP encoding, this article is for you.
- Good slides on “Explainable Machine Learning in NLP” from Peter Hase at UNC, well worth a read.
- You may have heard of a Kalman Filter... but what is it really and how does it work? Good sales pitch!
Surprisingly few software engineers and scientists seem to know about it, and that makes me sad because it is such a general and powerful tool for combining information in the presence of uncertainty. At times its ability to extract accurate information seems almost magical— and if it sounds like I’m talking this up too much, then take a look at this previously posted video where I demonstrate a Kalman filter figuring out the orientation of a free-floating body by looking at its velocity. Totally neat!
- One thing we all do on a regular basis is load up some data and then try and get a feel for it- how big, how many dimensions, what are the characteristics of and relationships between the dimensions etc etc. I normally just plug away in pandas, but there are now various elegant ‘profiling’ packages that do a lot of the work for you, well worth exploring:
- First of all pandas-profiling
- And the ‘new kid on the block’ lux
- Airflow is a great open source tool for scheduling and orchestration, well worth getting to know – an introduction here
- Useful lower level background on Deep Learning – understanding where to focus and what to focus on- from Horace He
- If you are investigating Deep Learning, it is increasingly likely you will be using PyTorch. This looks like a very useful add on for recommenders (TorchRec), and this ‘NN template‘ could be useful in setting up your PyTorch projects.
- This is very elegant – a visual introduction to machine learning

- Finally, an excellent review of ML Competitions over the last year across Kaggle and other platforms from newsletter subscribers Harald Carlens and Eniola Olaleye (shorter version here) – lots of great insight into the libraries and approaches used.
Practical tips
How to drive analytics and ML into production
- Lets start off with ‘friend of the section’ (well- he did give a fantastic fireside chat with us..) Andrew Ng discussing all things practical AI…
“In many industries where giant data sets simply don’t exist, I think the focus has to shift from big data to good data. Having 50 thoughtfully engineered examples can be sufficient to explain to the neural network what you want it to learn.”
- How should you structure your data team? One role that is often overlooked is the data product manager – good discussion on why this role is so important
- Ok… so you have your team setup, how should you run it? What principals should you adhere to? Great suggestions here (“0/1/Done Strategy”) from newsletter subscriber Marios Perrakis
- When you have models, pipelines and decision tools in production, being used across the organisation, you need to know they are working… or at least know when something has gone wrong. That is where ‘observability’ comes in – incredibly useful if you can get it right.
- Part of observability is understanding why something has changed. This is well worth a read- are there ways you can automatically explain changes in aggregations through ‘data-diff algorithms‘?
- How Netflix built their ‘trillions scale’ real time data platform
- We talk about MLOps on a reasonably regular basis – how best to implement, manage and monitor your machine learning models in production. Still struggling to figure out the right approach? You are definitely no the only one – “MLOps is a mess“
MLOps is in a wild state today with the tooling landscape offering more rare breeds than an Amazonian rainforest.
To give an example, most practitioners would agree that monitoring your machine learning models in production is a crucial part of maintaining a robust, performant architecture.
However when you get around to picking a provider I can name 6 different options without even trying: Fiddler, Arize, Evidently, Whylabs, Gantry, Arthur, etc. And we haven’t even mentioned the pure data monitoring tools.
Bigger picture ideas
Longer thought provoking reads – musing from some of the ‘OGs’ this month! – lean back and pour a drink!
- Whether or not AI systems are or ever can be truly ‘intelligent’, whether they really comprehend, even whether they have consciousness are very contentious questions… Mike Loukides kicks things off by digging into what we really mean by “understands”
"Comprehension is a poorly-defined term, like many terms that frequently show up in discussions of artificial intelligence: intelligence, consciousness, personhood. Engineers and scientists tend to be uncomfortable with poorly-defined, ambiguous terms. Humanists are not. My first suggestion is that these terms are important precisely because they’re poorly defined, and that precise definitions (like the operational definition with which I started) neuters them, makes them useless. And that’s perhaps where we should start a better definition of comprehension: as the ability to respond to a text or utterance."
- Love this … two of the biggest heavy hitters in the field getting straight to it on twitter (Ilya Sutskever = Chief Scientist at OpenAI, Yann LeCun = Chief Scientist at Meta/Facebook)
- Gary Marcus is well known for his scepticism on the future potential of Deep Learning. Here he digs into why
"To think that we can simply abandon symbol-manipulation is to suspend disbelief. "
- Another ‘heavy hitter’ Andrej Karpathy gives and excellent history lesson on NeuralNets and attempts to delve into what the future might bring
But the most important trend I want to comment on is that the whole setting of training a neural network from scratch on some target task (like digit recognition) is quickly becoming outdated due to finetuning, especially with the emergence of foundation models like GPT. These foundation models are trained by only a few institutions with substantial computing resources, and most applications are achieved via lightweight finetuning of part of the network, prompt engineering, or an optional step of data or model distillation into smaller, special-purpose inference networks
- Another take on where AI is heading this time from Jacob Steinhardt
- What does it mean for AI systems to be able build their own AI systems…
- An end to end exploration of what an ‘AI System’ really entails, digging into Amazon’s Alexa.
- And finally, a bit more esoteric … “musings on typicality” – worth a read and a cold cloth to the head!
To summarise: suppose you have an unfair coin that lands on heads 3 times out of 4. If you toss this coin 16 times, you would expect to see 12 heads (H) and 4 tails (T) on average. Of course you wouldn’t expect to see exactly 12 heads and 4 tails every time: there’s a pretty good chance you’d see 13 heads and 3 tails, or 11 heads and 5 tails. Seeing 16 heads and no tails would be quite surprising, but it’s not implausible: in fact, it will happen about 1% of the time. Seeing all tails seems like it would be a miracle. Nevertheless, each coin toss is independent, so even this has a non-zero probability of being observed.
If we do not ignore the order, and ask which sequence is the most likely, the answer is ‘all heads’. That may seem surprising at first, because seeing only heads is a relatively rare occurrence. But note that we’re asking a different question here, about the ordered sequences themselves, rather than about their statistics
Fun Practical Projects and Learning Opportunities
A few fun practical projects and topics to keep you occupied/distracted:
- I really like this – “If machine learning were around in the 1600s, would Newton have discovered his law of gravitation?” – Brilliant
- The weird and wonderful world of AI Art
- ‘What’s your favorite unpopular/forgotten Machine Learning method?’ with 260 comments….
- What happens when you don’t have the data? Most of the decisions we have to make on a day to day basis are done under uncertainty – how do we get better at dealing with this uncertainty and make better decisions? Practice – checkout Metaculus for a wide range of topical predictions you can get stuck into (hat-tip Ian Ozsvald)
- Finally, a bit of fun… how to wind up a statistician
"3. Treat research hypotheses like impressionist paintings
The big picture looks coherent but the details wash out when scrutinized. Use vague sciency sounding concepts that can mean anything.
Don't show it to the statistician until the end of the study. its best as a surprise"
Covid Corner
Apparently Covid is over – certainly there are very limited restrictions in the UK now
- However, no-one told the virus. The latest results from the ONS tracking study estimate 1 in 16 people (over 6%) in England have Covid. It’s worse in Scotland where the figure is 1 in 11. This is as bad as it has ever been in the whole 2+ years of the pandemic and a far cry from the 1 in 1000 we had last summer. Bear in mind in the chart below that the levels we had in February 2021 were enough to drive a national lockdown …
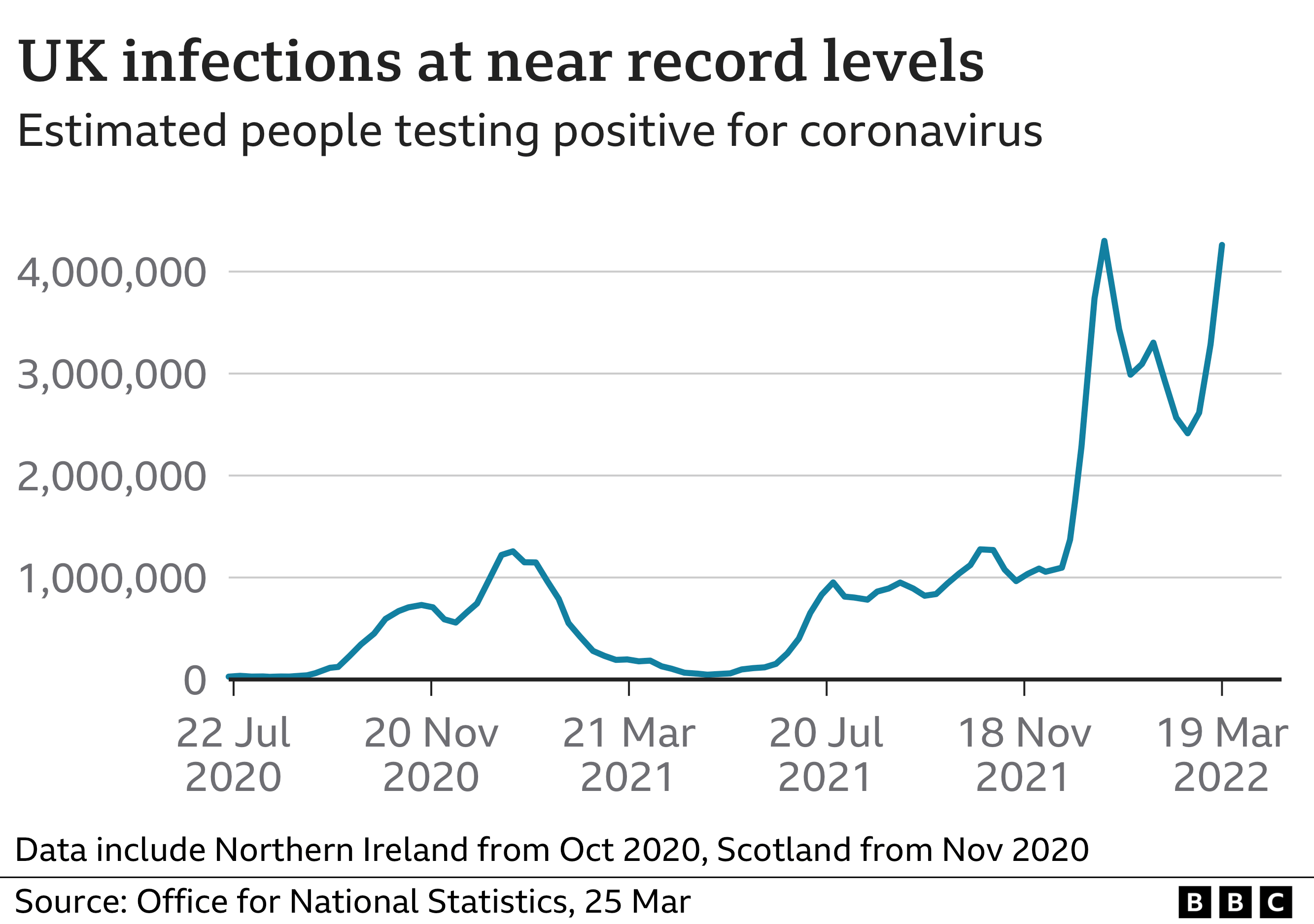
- And hospitalisations are now rising again as well – while we are removing all testing
- Good to see that scientists are still trying to understand the virus and its origins – and now have proven the original genetic link to the Huanan Seafood Market
- The RSS is running a series of ‘Covid Evidence Sessions’ to highlight important statistical and data issues that the upcoming public inquiries should look into.
Updates from Members and Contributors
- As highlighted in the practical tips section, Harald Carlens and Eniola Olaleye have finished their annual review of ML Competitions across Kaggle and other platforms, and written an excellent summary here (shorter version here)
- Also as highlighted above, Marios Perrakis recently published an article on implementing a novel operational strategy to delivering applied Data Science: “0/1/Done strategy” for Data Science
- Sarah Phelps is helping organise the first of this year’s ONS – UNECE Machine Learning Groups Coffee and Coding sessions, on 27 April 2022 at 0900 – 1100 and repeated at 1430 – 1630. This will be an interactive introductory session that discusses machine learning foundations and focuses on the theory behind these techniques. (Free registration using the links provided)
- Kevin O‘Brien calls our attention to the excellent PyDataGlobal organisation, and their upcoming PyData London conference (Fri 17th – Sun 19th June at the Tower Hotel, London). Still opportunities to present your work- if you are interested, submit here
- Glen Wright Colopy highlights a number of very relevant podcasts from the ASA (American Statistical Association)
- Our very own Martin Goodson: Practical Data Science & The UK’s AI Roadmap (as highlighted earlier)
- Chris Holmes: AI, Digital Health, & The Alan Turing Institute (digital health research at the Turing Institute plus Bayesian nonparametrics)
- Charlotte Deane: Bioinformatics, Deepmind’s AlphaFold 2, and Llamas (the role of statistical approaches to bioinformatics. Also integrating software engineers into academic research teams to better collaborate with industry)
Jobs!
A new section highlighting relevant job openings across the Data Science and AI community (let us know if you have anything you’d like to post here…)
- Holisticai, a startup focused on providing insight, assessment and mitigation of AI risk, has a number of relevant AI related job openings- see here for more details
- EvolutionAI, are looking for a machine learning research engineer to develop their award winning AI-powered data extraction platform, putting state of the art deep learning technology into production use. Strong background in machine learning and statistics required
- AstraZeneca are looking for a Data Science and AI Engagement lead – more details here
- Lloyds Register are looking for a data analyst to work across the Foundation with a broad range of safety data to inform the future direction of challenge areas and provide society with evidence-based information.
- Cazoo is looking for a number of senior data engineers – great modern stack and really interesting projects!
Again, hope you found this useful. Please do send on to your friends- we are looking to build a strong community of data science practitioners- and sign up for future updates here.
– Piers
The views expressed are our own and do not necessarily represent those of the RSS